Can I get there by model-ling?
Or is it much too near?
Welcome to part two of the two-part series on “Why is the GND in such a hurry?”. Last week, we established that, in fact, moving fast was kind of a good idea. Models prove it. But we were left a little dissatisfied, because the models didn’t really say much about whether moving fast was actually likely to happen. So, for inspiration, we looked to the past. We witnessed Sovacool’s (2016) examination of the historical evidence for rapid transitions and his subsequent appeal to the court of science to revise its earlier pessimistic verdict. Inspired, we (ok, I) then asked: couldn’t we use some of those insights from historical cases and infuse them into our models of the future? Couldn’t we, in other words, make our models be a little more social scientific?
Turns out, yes we can!
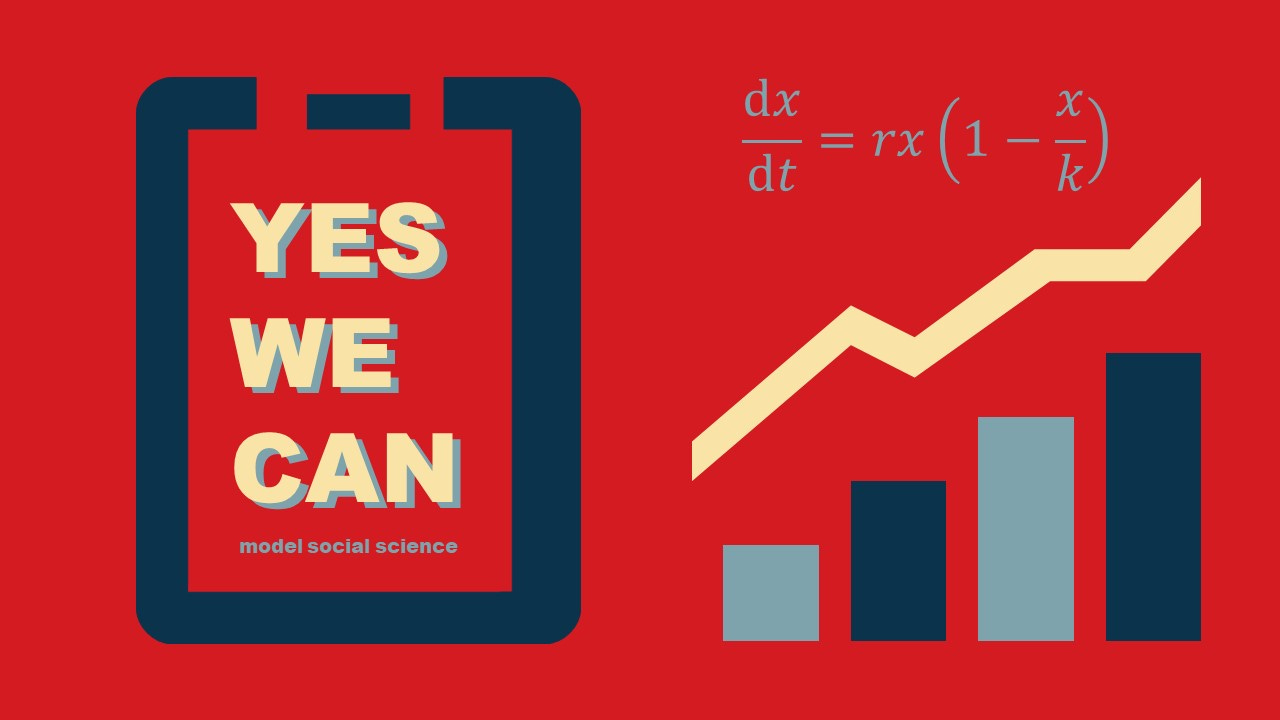
Below I present a handpicked selection of authors trying to re-write the models in just that way. By incorporating a more diverse set of sociological indicators they hope to be able to answer the question: ‘Under which societal conditions will we be able to meet our climate targets?’
iAM PROJECTING
Before diving into their models, let’s first take a look at the most influential models right now. The name of the game is Integrated Assessment Modelling (IAM). The ‘assessment’ part relates to the future state of greenhouse gas concentrations and/or global temperature. The ‘modelling’ means that we use quantitative proxies for real-world entities and drop these into statistical formulas meant to calculate the likelihood of said future state to occur (pause for breath). Finally, and this is where it gets interesting for us, integration refers to the attempt to combine societal developments with those from the natural world. The most famous organization using IAM is the UN’s International Panel on Climate Change (IPCC). It factors in such things as population, economic growth, and land use.
So, as you can see, there is already some attempt at a “sociology” in these models. In fact, the IPCC started to include more of these indicators in its models, because of the increasingly urgent imperative to spell out how we might obtain a bearable outcome and thus the need to talk more directly to policy makers (while still judiciously trying to avoid actually getting into policy and damaging its vital reputation of providing the facts and nothing but the facts).
The concept that the IPCC and many others use for this purpose is “Pathway”. It’s kind of a cool concept, in that it combines the idea of a path and a method, nicely embodied in one its definitions as a “course of action” (cf. Rosenbloom 2016: 39).
Taoist social science
Alright, now to recent social-science driven innovations. For example, a new approach to these Pathways has come up under the name of the Shared Socioeconomic Pathways (see this special issue). They continue the IPCC IAM tradition, but “[focus] on those elements of socioeconomic change that often cannot be covered by formal models” (Riahi et al 207: 156).
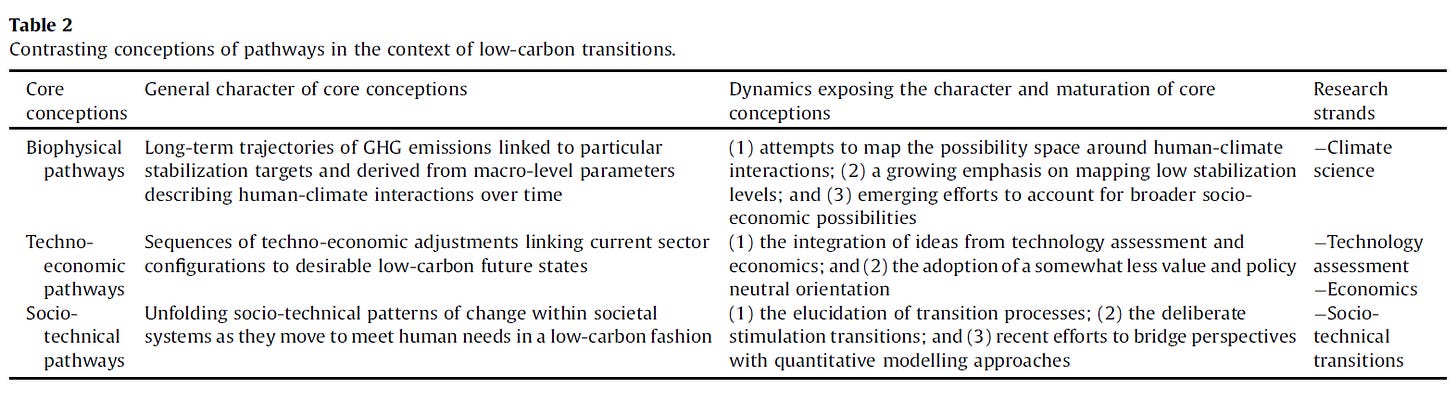
Rosenbloom (2017: 39) provides an overview of different disciplinary approaches to the idea of the pathway. In his categorization, the Socioeconomic Pathways are still part (to wit, #3) of the biophysical approach. See here for original.
Riahi et al (2017) explain the approach. They are also tackling this question ‘Under which conditions will we be able to meet our targets?’. To answer it, they try to quantify what might hurt (or help) attempts to reduce human impact on the climate (and to adapt to the climate’s impact on humans). Beyond the standard population and economic growth, they include such things as education levels and urbanization rates. (For example, they assume that as women become more educated, families will become smaller and fewer people will require carbon combustion to live wild and free.)
Now, all of their scenarios (sorry, “pathways”) assume that in the near term (until 2020), global initiatives to curb emissions will be scattered. In the ideal scenario (SSP1), everyone will then get together, heal the world and make it a better place, while in the worst-case scenario (SSP3), the world be rent apart by fights for parts of the ever-decreasing pie.
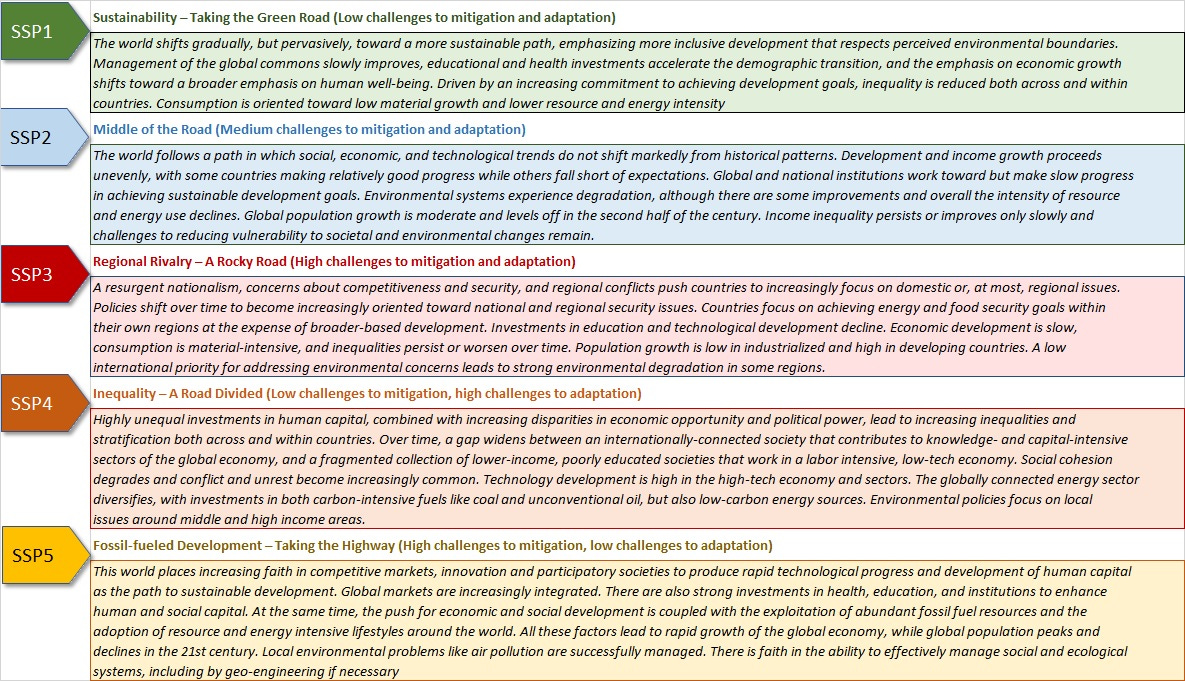
Summary of SSP pathway narratives. Taken from Riahi et al (2017: 157). See here for original.
In every scenario, the models want to provide insight in what climate policy can do, given certain socioeconomic developments. Thus, the models found that in SSP3 it was impossible for climate policy to make up for the lack of positive developments elsewhere in the world.
Others have since applied and updated these scenarios, such as Rogelj et al (2018), who wondered what world could manage to put a 1.5 degrees ceiling on climate warming. One of their results in particular is well worth pondering: in a world where social and geographical inequality reigns, a 1.5 degrees ceiling will be nigh impossible:
the lack of control over land-related emissions in developing countries and lower acceptability of CCS in developed countries in SSP4 make very low emissions pathways unachievable.
(Disclaimer: of course, this is still a modelled world. Real-world experiences may vary. I like Rogelj et al’s diplomatic phrasing here: “unsuccessful scenarios can be used as an indicator of infeasibility risk”.)
Of models and men
Speaking of models and the real world, these SSPs scenarios just the beginning of making projections cleave more faithfully to social reality. There is a growing – albeit still fledgling – body of literature in which scholars are either trying to update the input for the models, or change they very logic of the models in order to make them behave more like people would.
One call to action, penned by luminaries from both the forecasting and the transition studies branches of environmental policy (Turnheim et al 2015), is to integrate the projection and modelling techniques from forecasting science with both
the understanding of systemic momentum and inertia in (historical) transition studies, and
the lessons for change-on-the-ground from the sociology of the many pilot programs, living labs, and demonstration projects that we have (“initiative-based learning”).
Such a joint approach should help make scenarios and models more context-sensitive and capable of incorporating non-linear developments.
Yeah, about those non-linear developments. How come, Mercure et al (2016) wonder, when non-linearity is well-established in climate science, it hasn’t made its way into its models of human behaviour? One simple example of how one might simply incorporate them is by account for the famous “s curves” of increasing returns.
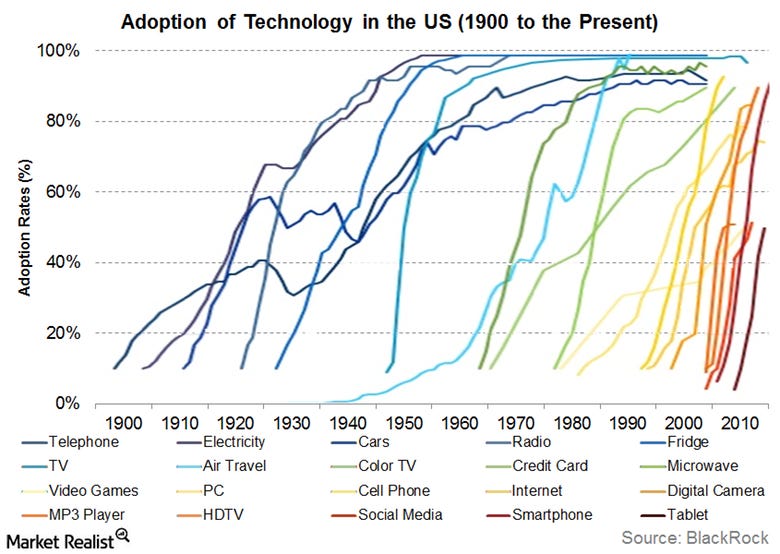
S-curves taken from David Roberts’ cautiously optimistic article
But non-linearity also allows for the concatenation or multiplication of uncertainties across different models. Thus, you could model different socially conditioned pathways to decarbonizing the electricity sector, but then the other is how those different pathways would interaction with climate pathways. Mercure et al. did some preliminary modelling, which allowed them to conclude that reducing carbon in the electricity sector by 90% is not enough for us to be more likely than 50% to stay under 2 degrees warming. Decarbonization needs to happen across sectors, notably transport (112). (Strategies to reduce energy demand will be a Very Important Topic in this newsletter.)
Finally, another particularly “unrealistic” assumption in IAM methodology is that of the rational agent. Take the purchase of electric vehicles. There are many peoples out there in the world, each with their own inclinations, contexts and capabilities. Current IA models do not account for such differences – they calculate future demand on the basis of GDP, prices, and a bunch of cost-calculations that are based on fully knowledgeable utility-cost maximizing ‘agents’, precisely the sort of creature that all of social science clearly says doesn’t exist.
So instead, McCollum et al (2016: 329ff.) tried to operationalize some variety in potential EV-drivers through three indicators: where people live (city, suburbs, countryside), if they tend to be early adopters, and how much they use their car. That is, everything but price-to-income ratios. In addition, they included “perceived downsides”, which they quantified into (so-called disutility) “costs”, such as the distance to nearest charging station (see p. 330 for details).
I’ll pick out one result: people are actually much less sensitive to carbon prices relative to other “costs” (actual or perceived) than assumed in existing IA models (that do not incorporate these others costs). In other words, you would need to jack up carbon prices (taxes) quite high in order to see significant effects on adoption of cleaner cars. Instead of raising carbon prices moderately and getting nothing in return, or raising them so high you’re pissing everyone off in the process, this result suggests a more effective policy approach would be to focus on reducing hurdles to adoption.
TBC IAM, SSP… TBC
I hope to have enlightened and entertained you with this brief foray into the art… ful science (phew) of projecting. Frankly, it stems more pessimistic than optimistic, because even though this newer literature might contain helpful pointers for policy makers, what these pointers point to is precisely the comprehensive and socially sensitive policy that will be hardest to get through the political process and into organizational routines. Let me know if you have evidence to the contrary!
I will revisit this rapidly evolving field now and again. For the next few issues, I will be zooming back in on specific issues that the GND has put on the agenda, hopefully generating more vivid and concrete signposts!
Sources
McCollum, David L., Charlie Wilson, Hazel Pettifor, Kalai Ramea, Volker Krey, Keywan Riahi, Christoph Bertram, Zhenhong Lin, Oreane Y. Edelenbosch, and Sei Fujisawa. 2017. "Improving the behavioral realism of global integrated assessment models: An application to consumers’ vehicle choices". Transportation Research Part D: Transport and Environment. 55: 322-342. http://dx.doi.org/10.1016/j.trd.2016.04.003
Mercure, J.F.A., H. Pollitt, A.M. Bassi, J.E. Viñuales, and N.R. Edwards. 2016. "Modelling complex systems of heterogeneous agents to better design sustainability transitions policy". Global Environmental Change-Human Policy Dimensions. 37: 102-115. (Open Access) http://dx.doi.org/10.1016/j.gloenvcha.2016.02.003 (For a quick and dirty overview of the problems with ‘traditional’ IAM, check out the first section of this article, p. 103ff.)
Riahi, Keywan, Detlef P. van Vuuren, Elmar Kriegler, Jae Edmonds, Brian C. O’Neill, Shinichiro Fujimori, Nico Bauer, et al. 2017. "The Shared Socioeconomic Pathways and their energy, land use, and greenhouse gas emissions implications: An overview". Global Environmental Change. 42: 153-168. http://dx.doi.org/10.1016/j.gloenvcha.2016.05.009 (Open Access)
Rogelj J., Fujimori S., Hasegawa T., Krey V., Riahi K., Fricko O., et al. 2018. "Scenarios towards limiting global mean temperature increase below 1.5 °c". Nature Climate Change. 8 (4): 325-332. https://doi.org/10.1038/s41558-018-0091-3
Rosenbloom, Daniel. 2017. "Pathways: An emerging concept for the theory and governance of low-carbon transitions". Global Environmental Change. 43: 37-50. http://dx.doi.org/10.1016/j.gloenvcha.2016.12.011
Turnheim B., Berkhout F., Geels F., et al. 2015. "Evaluating sustainability transitions pathways: Bridging analytical approaches to address governance challenges". Global Environmental Change. 35: 239-253. http://dx.doi.org/10.1016/j.gloenvcha.2015.08.010
Sovacool, Benjamin K. 2016. "How long will it take? Conceptualizing the temporal dynamics of energy transitions". Energy Research & Social Science. 13: 202-215. http://dx.doi.org/10.1016/j.erss.2015.12.020
If you do not have the appropriate credentials to cross the paywall to these articles, maybe you can check out sci-hub.tw (just type in the doi number), or if you are uncomfortable with that, send me a message and I’ll lend you a copy.